Course Content
Probability Theory Mastering
1. Additional Statements From The Probability Theory
3. Estimation of Population Parameters
4. Testing of Statistical Hypotheses
Probability Theory Mastering
Characteristics of Random Variables
Characteristics of random variables are important because they provide a formal way to describe and analyse the behaviour of uncertain events and outcomes in a probabilistic framework. They allow us to quantify and measure the uncertainty, variability, and central tendency of random variables, which are essential for making informed decisions and drawing meaningful conclusions from data.
The probability distribution of a random variable
The probability distribution of a random variable specifies the probabilities associated with each possible value in its domain. It can be represented using Probability Mass Function (PMF) for discrete random variables, or the Probability Density Function (PDF) for continuous random variables. We considered PMF and PDF in the previous chapter.
Let's look at the PDF of some continuous distributions:
Expected value
The expected value, also known as the mean or average, of a random variable is a measure of the central tendency of the random variable. It represents the weighted average of all possible values of the random variable, weighted by their respective probabilities.
Assume that X is discrete random variable and it's PMF looks like:
Values | x_1 | x_2 | x_3 | .... | x_N |
Probability | p_1 | p_2 | p_3 | .... | p_N |
We can calculate expectation as follows:
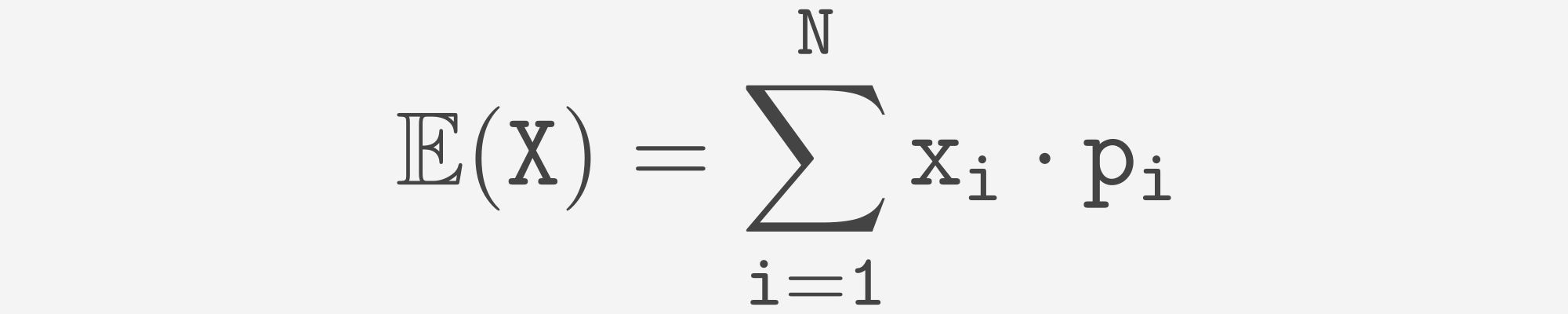
Now let's calculate expectation for continuous random variable X. Assuming, that f(x)
if a PDF of this variable we can calculate expectation as follows:
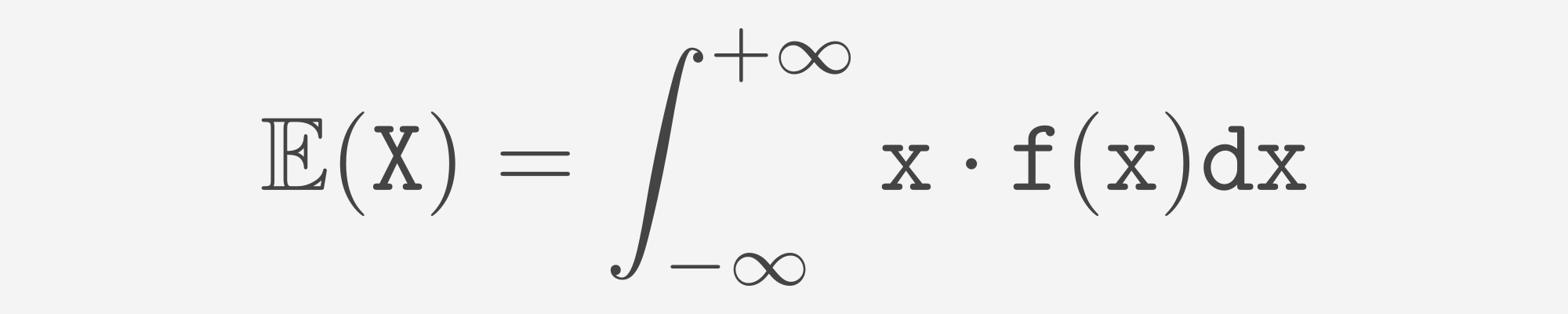
Let's look at the PDF plot of normal distribution with different means:
Variance
The variance of a random variable is a measure of the dispersion or spread of the values of the random variable around its expected value. It quantifies the variability or uncertainty associated with the random variable. To calculate variance, we can use the following formula:
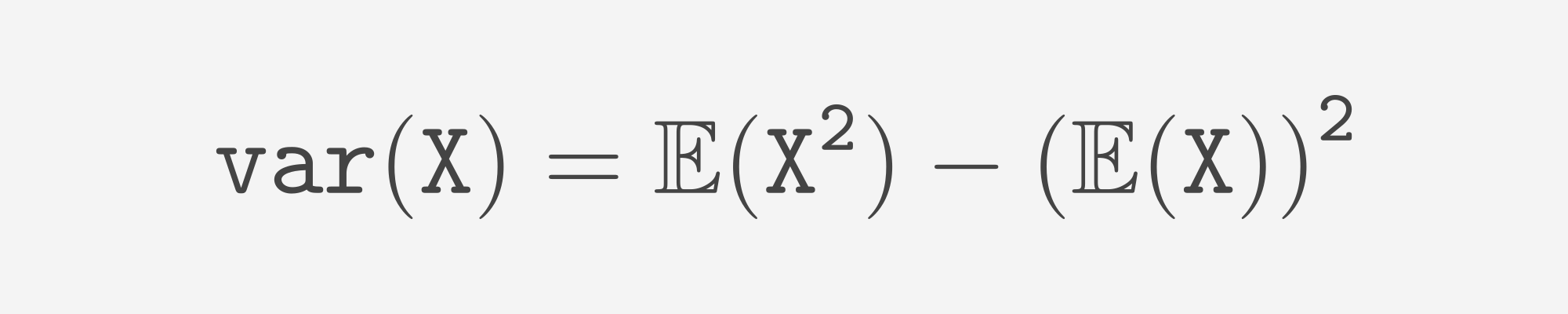
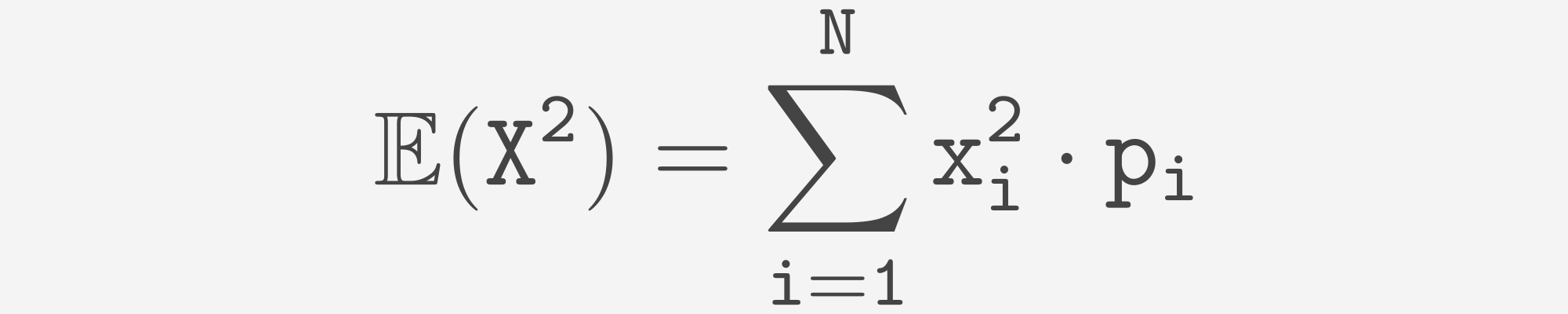
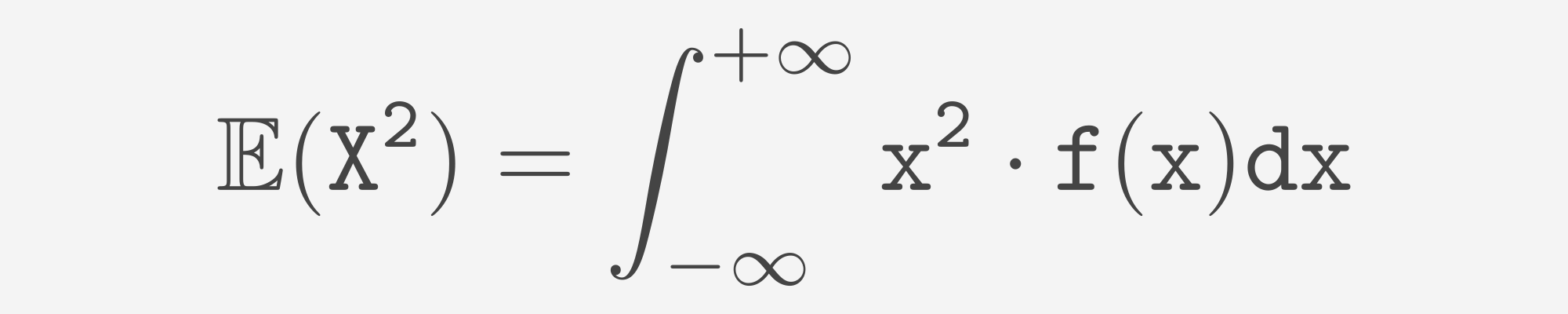
Let's look at the PDF plot of the normal distribution with different variances and fixed mean:
The square root of the variance is called standard deviation. Using standard deviation instead of variance can be advantageous due to two factors:
- We will work with smaller absolute values (while the variance will be, for example, 225, the standard deviation will be only 15, which is much more convenient in terms of calculations);
- The standard deviation is measured in the same units as the data, which can be important in certain cases (if, for example, we work with length in meters, then the variance will be measured in square meters, while the standard deviation is still in meters).
Note
The
scale
keyword of thescipy.stats.norm
class represents the standard deviation of the normal distribution.
Theloc
keyword of thescipy.stats.norm
class represents the mean of the normal distribution.
Median
The median measures the central tendency in statistics that represents the middle value in a dataset when arranged in ascending or descending order.
We can calculate the median of random variable X as follows:
- Determine the CDF of
X
; - Find the value y such that CDF(
y
) =0.5
; - This value y is the median of the random variable
X
.
It's important to understand that expected value and median are two different characteristics: expected value is the weighted average of all possible values of the random variable, whereas the weights are the probabilities of those values occurring; on the other hand, the median is the value that separates the data into two halves.
For random variables with skewed distributions, this difference is the most significant.
Let's look at the example below:
We see that the expected value is shifted in the direction of the tail of the distribution. We have to admit that the expected value is more affected by outliers and anomalies, which makes this characteristic less reliable for real data analytics.
Everything was clear?
Course Content
Probability Theory Mastering
1. Additional Statements From The Probability Theory
3. Estimation of Population Parameters
4. Testing of Statistical Hypotheses
Probability Theory Mastering
Characteristics of Random Variables
Characteristics of random variables are important because they provide a formal way to describe and analyse the behaviour of uncertain events and outcomes in a probabilistic framework. They allow us to quantify and measure the uncertainty, variability, and central tendency of random variables, which are essential for making informed decisions and drawing meaningful conclusions from data.
The probability distribution of a random variable
The probability distribution of a random variable specifies the probabilities associated with each possible value in its domain. It can be represented using Probability Mass Function (PMF) for discrete random variables, or the Probability Density Function (PDF) for continuous random variables. We considered PMF and PDF in the previous chapter.
Let's look at the PDF of some continuous distributions:
Expected value
The expected value, also known as the mean or average, of a random variable is a measure of the central tendency of the random variable. It represents the weighted average of all possible values of the random variable, weighted by their respective probabilities.
Assume that X is discrete random variable and it's PMF looks like:
Values | x_1 | x_2 | x_3 | .... | x_N |
Probability | p_1 | p_2 | p_3 | .... | p_N |
We can calculate expectation as follows:
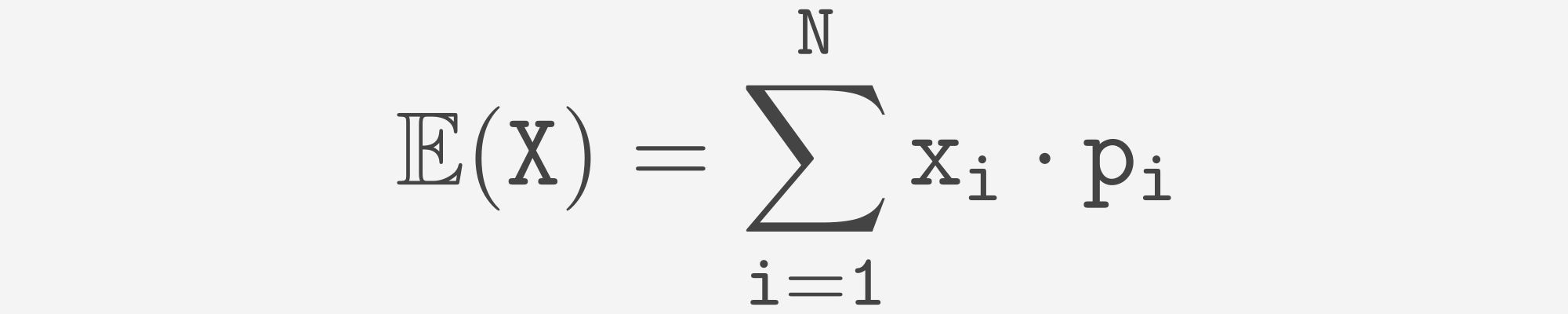
Now let's calculate expectation for continuous random variable X. Assuming, that f(x)
if a PDF of this variable we can calculate expectation as follows:
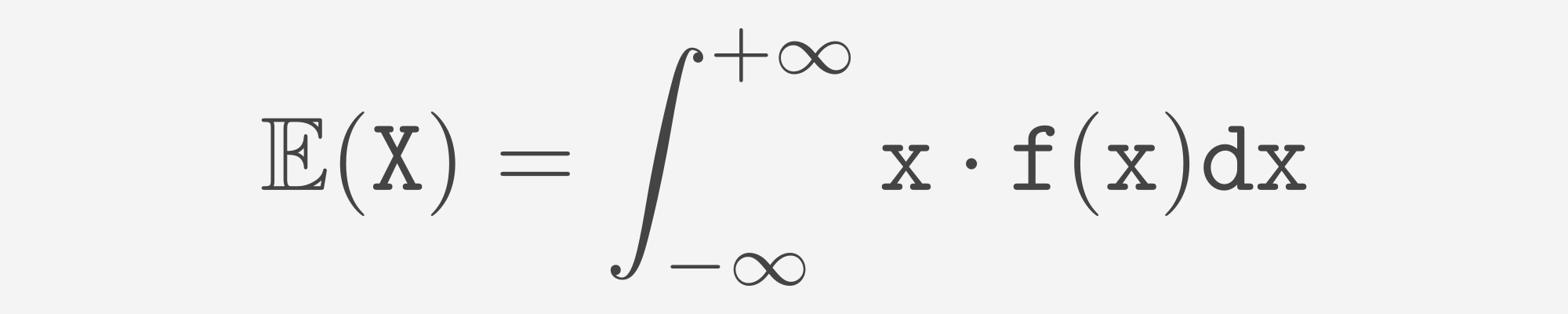
Let's look at the PDF plot of normal distribution with different means:
Variance
The variance of a random variable is a measure of the dispersion or spread of the values of the random variable around its expected value. It quantifies the variability or uncertainty associated with the random variable. To calculate variance, we can use the following formula:
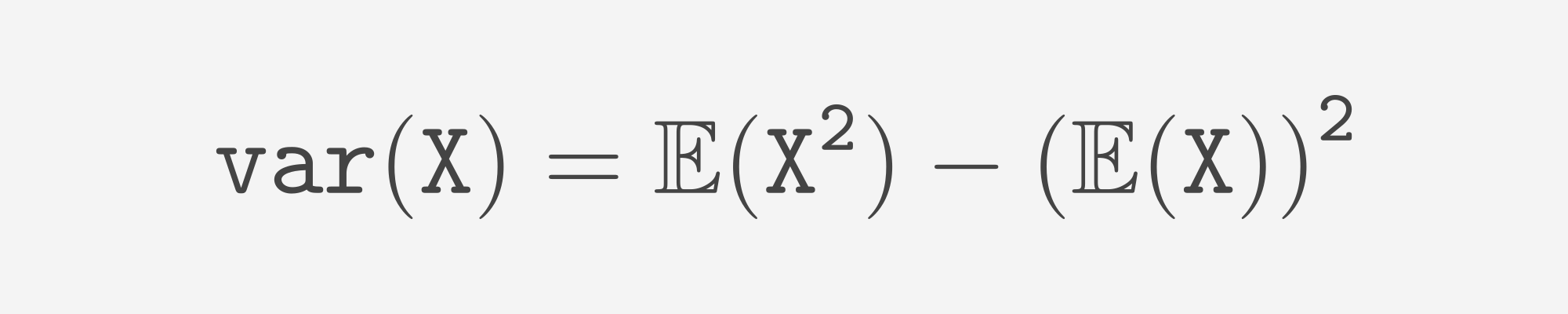
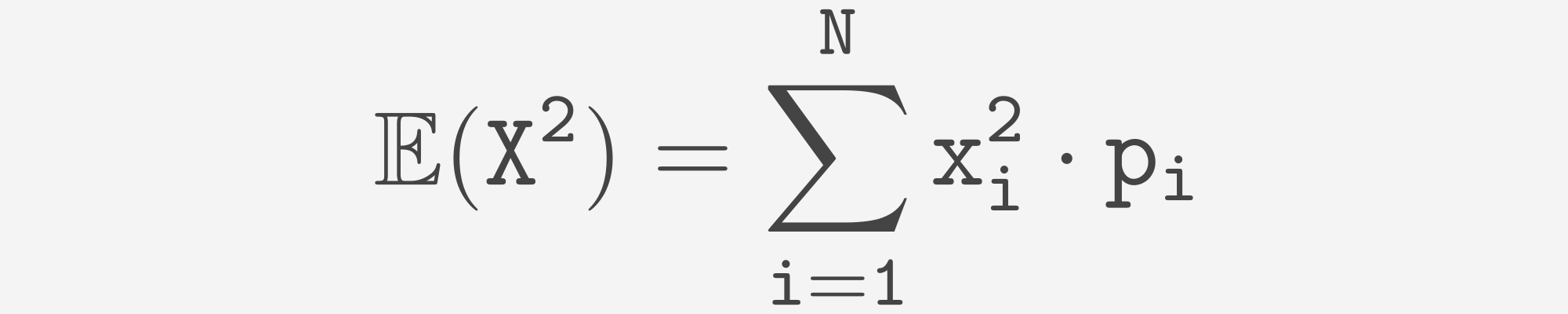
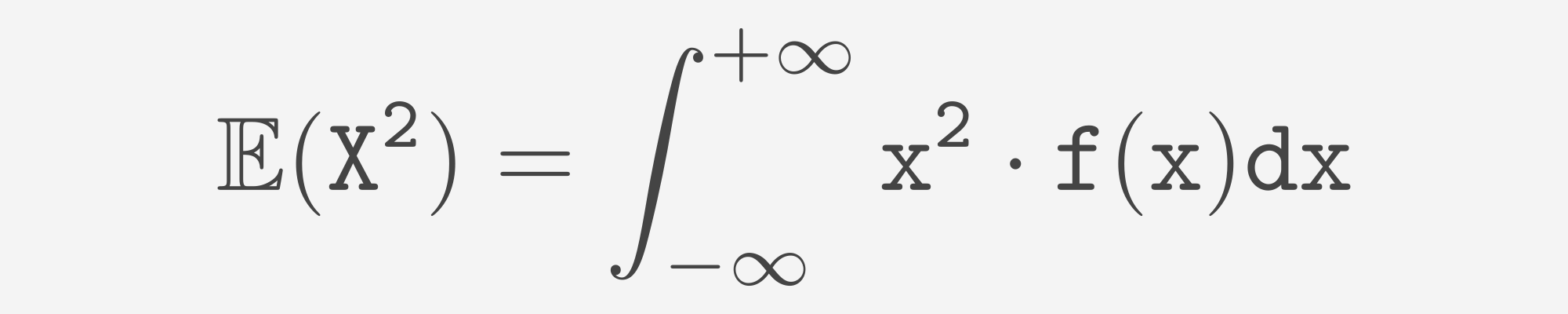
Let's look at the PDF plot of the normal distribution with different variances and fixed mean:
The square root of the variance is called standard deviation. Using standard deviation instead of variance can be advantageous due to two factors:
- We will work with smaller absolute values (while the variance will be, for example, 225, the standard deviation will be only 15, which is much more convenient in terms of calculations);
- The standard deviation is measured in the same units as the data, which can be important in certain cases (if, for example, we work with length in meters, then the variance will be measured in square meters, while the standard deviation is still in meters).
Note
The
scale
keyword of thescipy.stats.norm
class represents the standard deviation of the normal distribution.
Theloc
keyword of thescipy.stats.norm
class represents the mean of the normal distribution.
Median
The median measures the central tendency in statistics that represents the middle value in a dataset when arranged in ascending or descending order.
We can calculate the median of random variable X as follows:
- Determine the CDF of
X
; - Find the value y such that CDF(
y
) =0.5
; - This value y is the median of the random variable
X
.
It's important to understand that expected value and median are two different characteristics: expected value is the weighted average of all possible values of the random variable, whereas the weights are the probabilities of those values occurring; on the other hand, the median is the value that separates the data into two halves.
For random variables with skewed distributions, this difference is the most significant.
Let's look at the example below:
We see that the expected value is shifted in the direction of the tail of the distribution. We have to admit that the expected value is more affected by outliers and anomalies, which makes this characteristic less reliable for real data analytics.
Everything was clear?