Зміст курсу
Cluster Analysis in Python
Cluster Analysis in Python
How Similar are the Results?
Well done! Let's look at the last line charts you built in the previous chapter.
As you can see, only the ward linkage could catch the 'downward up to July' trend. Both results are different. But let's find out how different they are using the rand index.
Завдання
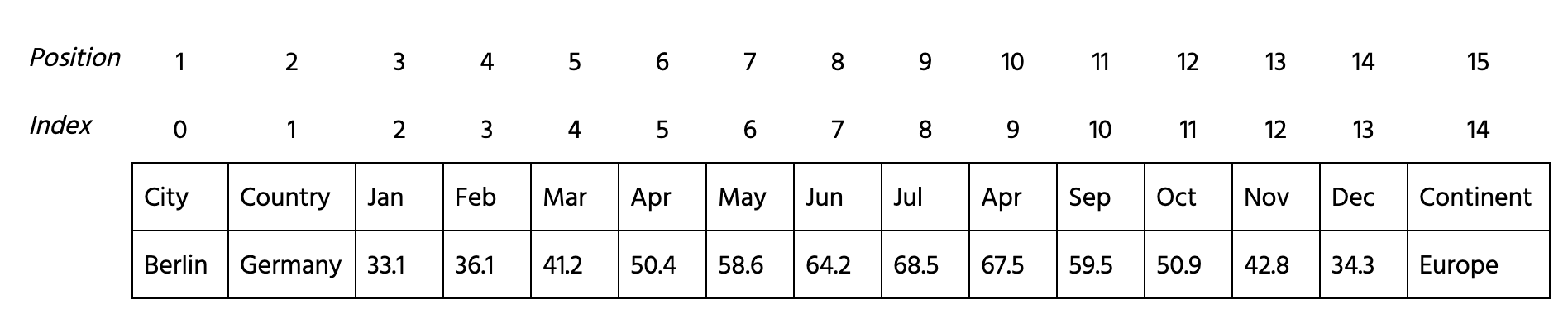
Compute the rand index to compare the results of using complete and ward linkages. Follow the next steps:
- Import functions needed:
rand_score
fromsklearn.metrics
.AgglomerativeClustering
fromsklearn.cluster
.
- Create two models
model_complete
andmodel_ward
performing a hierarchical clustering with 4 clusters both and'complete'
and'ward'
linkages respectively. - Fit the 3-14 columns of
data
to models and predict the labels. Save the labels formodel_complete
withinlabels_complete
and formodel_ward
withinlabels_ward
. - Compute the rand index using
labels_complete
andlabels_ward
.
Завдання
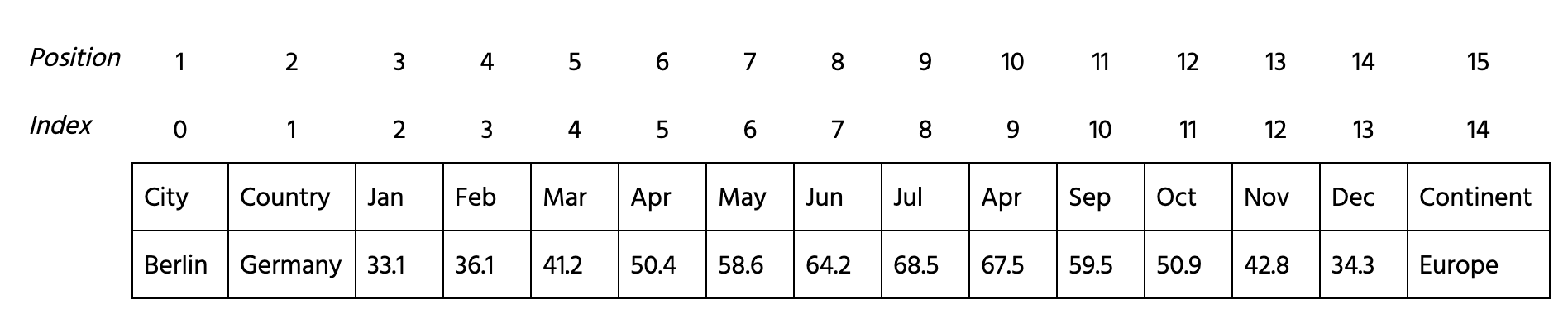
Compute the rand index to compare the results of using complete and ward linkages. Follow the next steps:
- Import functions needed:
rand_score
fromsklearn.metrics
.AgglomerativeClustering
fromsklearn.cluster
.
- Create two models
model_complete
andmodel_ward
performing a hierarchical clustering with 4 clusters both and'complete'
and'ward'
linkages respectively. - Fit the 3-14 columns of
data
to models and predict the labels. Save the labels formodel_complete
withinlabels_complete
and formodel_ward
withinlabels_ward
. - Compute the rand index using
labels_complete
andlabels_ward
.
Все було зрозуміло?
How Similar are the Results?
Well done! Let's look at the last line charts you built in the previous chapter.
As you can see, only the ward linkage could catch the 'downward up to July' trend. Both results are different. But let's find out how different they are using the rand index.
Завдання
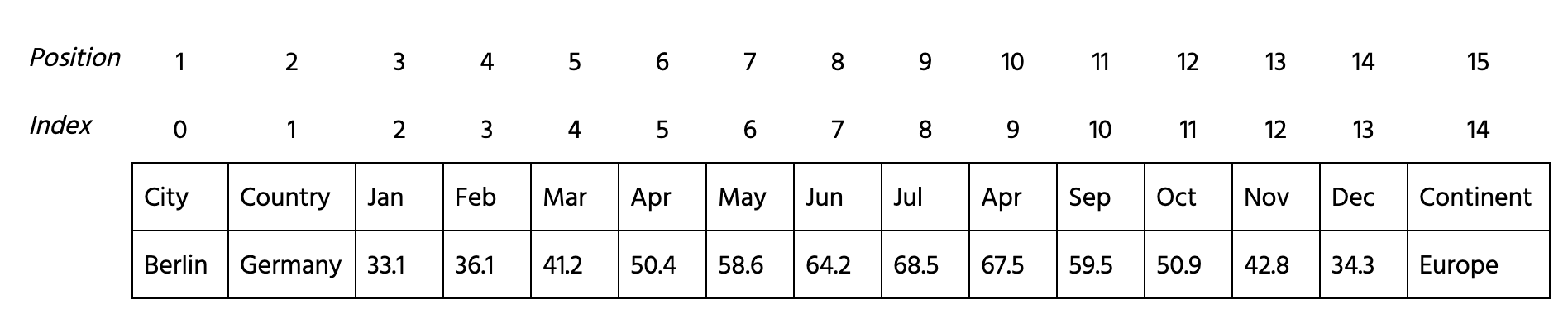
Compute the rand index to compare the results of using complete and ward linkages. Follow the next steps:
- Import functions needed:
rand_score
fromsklearn.metrics
.AgglomerativeClustering
fromsklearn.cluster
.
- Create two models
model_complete
andmodel_ward
performing a hierarchical clustering with 4 clusters both and'complete'
and'ward'
linkages respectively. - Fit the 3-14 columns of
data
to models and predict the labels. Save the labels formodel_complete
withinlabels_complete
and formodel_ward
withinlabels_ward
. - Compute the rand index using
labels_complete
andlabels_ward
.
Завдання
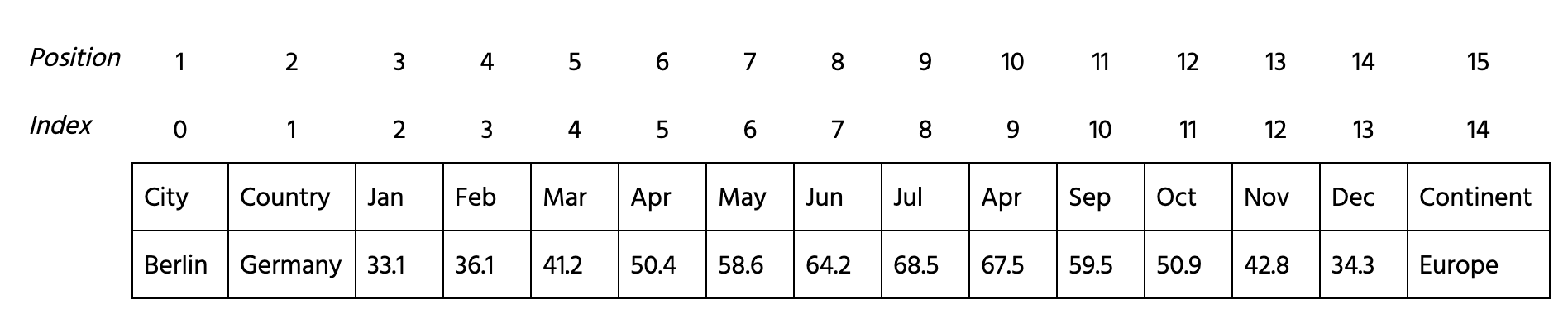
Compute the rand index to compare the results of using complete and ward linkages. Follow the next steps:
- Import functions needed:
rand_score
fromsklearn.metrics
.AgglomerativeClustering
fromsklearn.cluster
.
- Create two models
model_complete
andmodel_ward
performing a hierarchical clustering with 4 clusters both and'complete'
and'ward'
linkages respectively. - Fit the 3-14 columns of
data
to models and predict the labels. Save the labels formodel_complete
withinlabels_complete
and formodel_ward
withinlabels_ward
. - Compute the rand index using
labels_complete
andlabels_ward
.
Все було зрозуміло?
How Similar are the Results?
Well done! Let's look at the last line charts you built in the previous chapter.
As you can see, only the ward linkage could catch the 'downward up to July' trend. Both results are different. But let's find out how different they are using the rand index.
Завдання
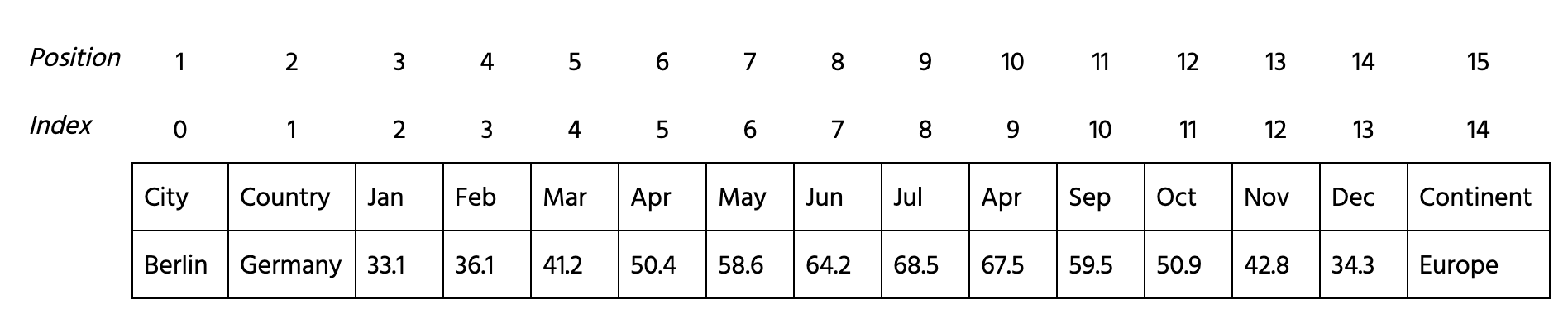
Compute the rand index to compare the results of using complete and ward linkages. Follow the next steps:
- Import functions needed:
rand_score
fromsklearn.metrics
.AgglomerativeClustering
fromsklearn.cluster
.
- Create two models
model_complete
andmodel_ward
performing a hierarchical clustering with 4 clusters both and'complete'
and'ward'
linkages respectively. - Fit the 3-14 columns of
data
to models and predict the labels. Save the labels formodel_complete
withinlabels_complete
and formodel_ward
withinlabels_ward
. - Compute the rand index using
labels_complete
andlabels_ward
.
Завдання
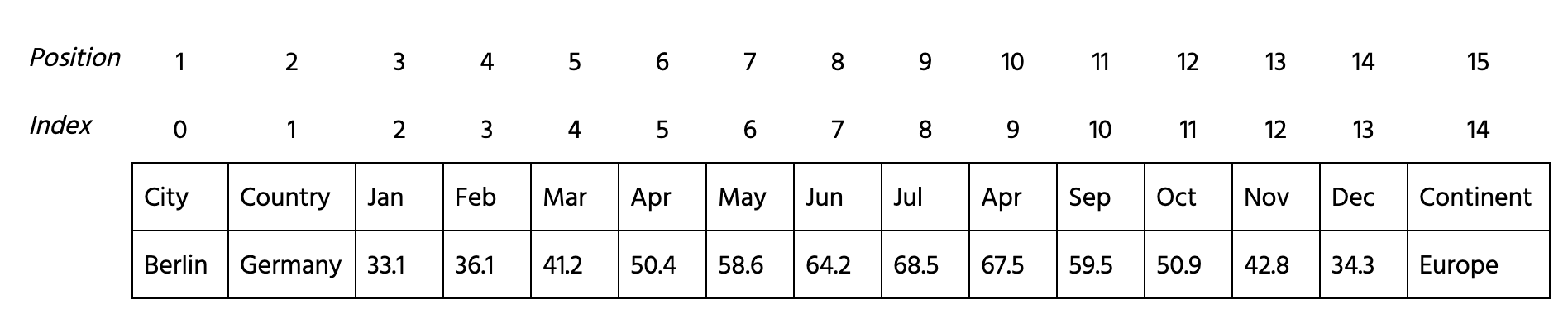
Compute the rand index to compare the results of using complete and ward linkages. Follow the next steps:
- Import functions needed:
rand_score
fromsklearn.metrics
.AgglomerativeClustering
fromsklearn.cluster
.
- Create two models
model_complete
andmodel_ward
performing a hierarchical clustering with 4 clusters both and'complete'
and'ward'
linkages respectively. - Fit the 3-14 columns of
data
to models and predict the labels. Save the labels formodel_complete
withinlabels_complete
and formodel_ward
withinlabels_ward
. - Compute the rand index using
labels_complete
andlabels_ward
.
Все було зрозуміло?
Well done! Let's look at the last line charts you built in the previous chapter.
As you can see, only the ward linkage could catch the 'downward up to July' trend. Both results are different. But let's find out how different they are using the rand index.
Завдання
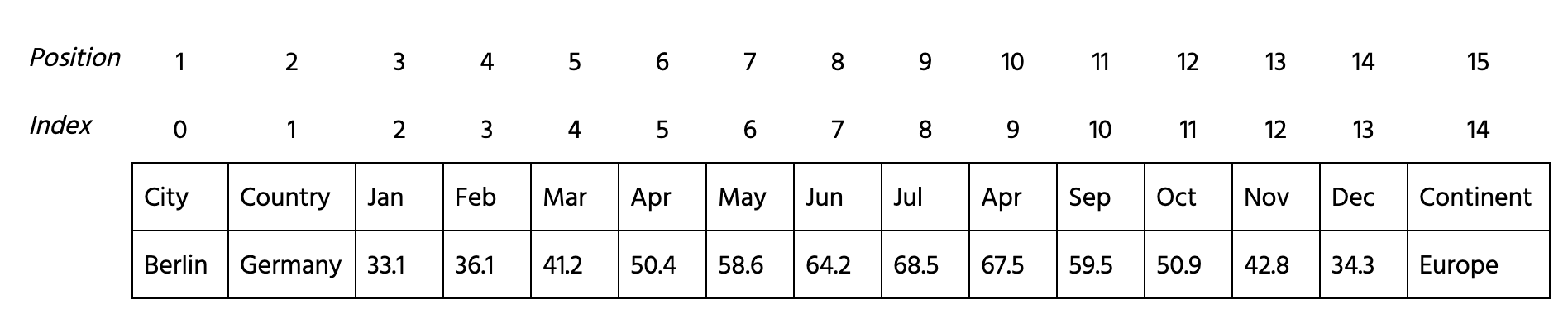
Compute the rand index to compare the results of using complete and ward linkages. Follow the next steps:
- Import functions needed:
rand_score
fromsklearn.metrics
.AgglomerativeClustering
fromsklearn.cluster
.
- Create two models
model_complete
andmodel_ward
performing a hierarchical clustering with 4 clusters both and'complete'
and'ward'
linkages respectively. - Fit the 3-14 columns of
data
to models and predict the labels. Save the labels formodel_complete
withinlabels_complete
and formodel_ward
withinlabels_ward
. - Compute the rand index using
labels_complete
andlabels_ward
.